A machine learning-based model developed by a team of researchers at the University of Lille in Lille, Hauts-de-France, France, can predict individual weight-loss trajectories up to five years after bariatric surgery simultaneously for RYGB (Roux-en-Y gastric bypass), SG (sleeve gastrectomy) and AGB (adjustable gastric banding).
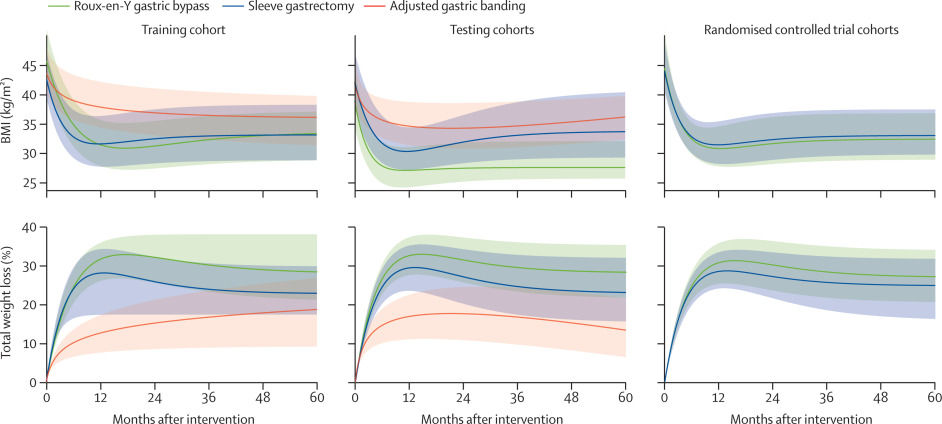
According to the study, ‘Development and validation of an interpretable machine learning-based calculator for predicting 5-year weight trajectories after bariatric surgery: a multinational retrospective cohort SOPHIA study’, published in The Lancet Digital Health journal, the model is internationally validated, and is the first to provide preoperative predictions of weight trajectories up to five years after surgery.
It is difficult to forecast weight loss outcomes following bariatric surgery as weight loss can change over time and varies between procedures and individuals. However, by estimating expected weight loss trajectory after surgery can help inform clinical decisions by patients and their health-care providers.
Machine learning methods have the potential to discover subtle, non-linear patterns in data that are often not accessible using traditional approaches such as logistic regression, and machine learning models have outperformed logistic regression using National Surgical Quality Improvement Program data.
For this multinational retrospective observational study, the researchers enrolled adult participants (aged ≥18 years) from ten prospective cohorts (including ABOS [NCT01129297], BAREVAL [NCT02310178], the Swedish Obese Subjects study, and a large cohort from the Dutch Obesity Clinic [Nederlandse Obesitas Kliniek]) and two randomised trials (SleevePass [NCT00793143] and SM-BOSS [NCT00356213]) in Europe, the Americas, and Asia, with afive5 year follow-up after RYYGB, SG amd AGB. The primary outcome was BMI at five years. A model was developed using least absolute shrinkage and selection operator to select variables and the classification and regression trees algorithm to build interpretable regression trees. The performances of the model were assessed through the median absolute deviation (MAD) and root mean squared error (RMSE) of BMI.
In total, 10,231 patients from 12 centres in ten countries were included in the analysis, corresponding to 30,602 patient-years. Among participants in all 12 cohorts, 7,701 (75.3%) were female, 2,530 (24.7%) were male. Among 434 baseline attributes available in the training cohort, seven variables were selected: height, weight, intervention type, age, diabetes status, diabetes duration, and smoking status.
At five years, across external testing cohorts the overall mean MAD BMI was 2.8 kg/m2 (95% CI 2.6–3.0) and mean RMSE BMI was 4.7 kg/m2 (4.4–5.0), and the mean difference between predicted and observed BMI was –0.3 kg/m2 (SD 4.7). This model is incorporated in an easy to use and interpretable web-based prediction tool to help inform clinical decision before surgery, the authors noted.
The model was developed using machine-learning approaches, notably LASSO and CART algorithms. These methods enable the identification of non-linear relationships and non-parametric models, offering a more comprehensive and accurate understanding of weight loss prediction, the researchers added.
“In summary, we have developed and validated an easy-to-use and interpretable model that provides individual predictions of weight loss trajectory after bariatric surgery. We have shown its generalisability and transportability across multiple cohorts in Europe, the Americas, and Asia, as well as its performance in intervention clinical trials,” the authors concluded. “Individual weight loss trajectory prediction appears to be an accurate and simple strategy to inform clinical decisions for both health-care providers and patients before surgery. Our model can also be used postoperatively to identify patients whose actual weight loss trajectories differ from their predicted trajectory, thus allowing the timely implementation of appropriate clinical interventions.”
To access this paper, please click here
Comentários