3D imaging and AI provides more accurate assessments of body fat and muscle distribution
- owenhaskins
- Feb 4
- 2 min read
A recent study that used an innovative method for analysing body composition using advanced 3D imaging and deep learning techniques, has concluded that this approach provides more accurate assessments of body fat and muscle distribution, which are crucial for understanding health risks associated with various conditions.
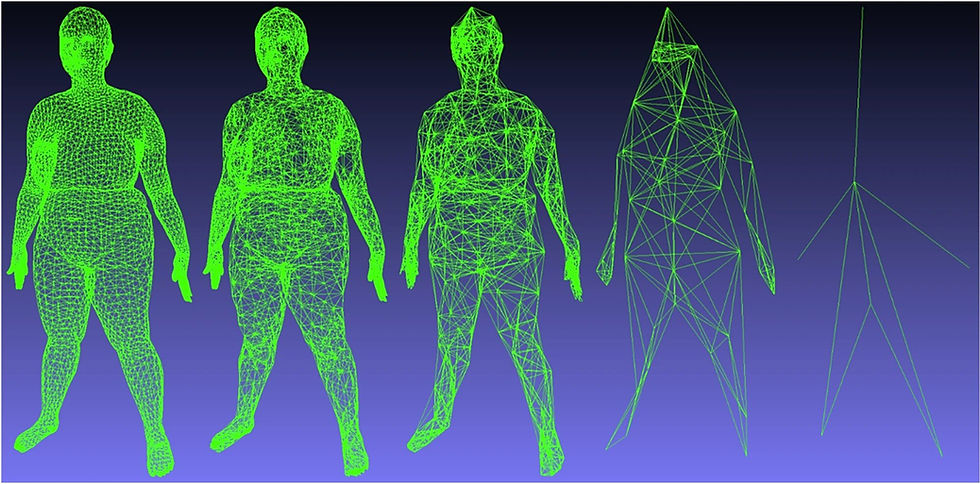
Researchers from Pennington Biomedical Research Center, University of Washington, University of Hawaii and University of California-San Francisco used deep, nonlinear methods to enhance the estimation of body composition parameters, surpassing the accuracy of previous linear models. This advancement holds potential for improving assessments in clinical settings and research applications.
Key highlights of the study include:
Advanced imaging: The researchers utilised 3D imaging technology to capture detailed representations of the body's shape.
Deep learning application: By applying sophisticated deep learning algorithms, the study achieved more precise estimations of body composition compared to traditional methods.
Health implications: Accurate body composition analysis is essential for assessing health risks related to obesity, cardiovascular diseases, and other metabolic disorders.
"Dr Steven Heymsfield has extensive experience in human obesity, energy balance regulation, and the development of methods for evaluating body composition," said Dr John Kirwan, Pennington Biomedical Executive Director. "His contributions to this field have been pivotal in advancing the understanding of human metabolism and the application of new technologies such as 3D optical imaging in medical research."
This development represents a step forward in medical imaging and health assessment, offering a more reliable tool for clinicians and researchers to evaluate body composition and associated health risks.
"To easily and quickly create a detailed digital map of a person's body shape and then to use that information to generate not only accurate estimates of their body composition and health risks, but also corresponding 3D images, was almost unimaginable just a few years ago," said Dr Heymsfield, Professor of Metabolism & Body Composition at Pennington Biomedical. "Technological advances like this require skills from a broad range of scientists and I'm pleased to have the opportunity here at Pennington Biomedical to work with colleagues from across the country and the world."
The findings were reported in the paper, ‘3D convolutional deep learning for nonlinear estimation of body composition from whole body morphology’, published in npj Digital Medicine. To access this paper, please click here
Komentarze